HAPPE AI
(Pronounced “happy”)
A better, happier you is just a step away…
WHY HAPPE AI IS DIFFERENT
- All wellness sessions are interactive, two-way conversations
- A live therapist controls every conversation with the help of AI
- Rule-based responses ensures there are no AI hallucinations
- Affordable Pricing: Just $4 per 15-minute session
- Wellness support sessions are interactive two-way text or
voice conversations available 24×7 in multiple languages
JOIN OUR CAUSE
We’re seeking behavioral health leaders and those in academia to help drive our mission of providing accessible and affordable wellness support.
Voice-Based Wellness Support
The accuracy of AI in detecting voice-based emotions without relying on keywords is a complex matter and depends heavily on the specific technologies, training datasets, and context. Here are the key factors and general estimates:
1. General Accuracy Range
- Current Accuracy
- Most advanced voice emotion recognition models achieve 70–85% accuracy in controlled environments.
- Accuracy in natural, complex conversations like therapy sessions drops to 50–70%, due to subtleties in emotional expression and environmental noise.
- Contextual Emotion Detection
AI is less reliable when emotions are inferred solely from nuanced behaviors like response delays, pitch variation, or speech rate changes without linguistic or context cues. Accuracy in these cases can drop to 40–60%.
2. Challenges in High Accuracy
- Ambiguity in Responses: Emotional cues like pauses or pitch changes can be ambiguous. For example, a pause might signal sadness, but it could also indicate deep thought.
- Individual Variation: Emotional expression varies significantly between individuals due to culture, personality, and context. A pause for one person may not carry the same emotional weight for another.
- Overlapping Signals: Mixed emotions (e.g., sadness and anxiety) can confuse models, leading to reduced accuracy.
- No Clear Ground Truth: Unlike facial expressions, there’s no universally agreed-upon mapping of specific voice patterns to emotions.
3. Improving Accuracy
- Advanced Models: Deep learning models trained on large, diverse datasets can achieve up to 75–85% accuracy in specific scenarios, but this requires robust input data and careful tuning.
- Multimodal Analysis: Combining voice analysis with additional data streams (e.g., facial expressions, physiological sensors) boosts accuracy to 85–90%.
- Context Incorporation: Systems that integrate contextual awareness (e.g., the ongoing therapy topic, historical behavior patterns) can outperform basic voice-only systems.
4. Realistic Expressions In Therapy Settings
In a therapy-like conersation
- AI could detect general emotional states(e.g., anxiuos, sad, calm) with 50-70% accuracy without keywords.
- For nuanced interpretations like detecting anxiety based on a 15% faster response or sadness from slower speech, accuracy could be closer to 40-50%, unless the AI is highly trained on therapy-specific datasets.
5. What Affects Accuracy?
- Training Data Quality: Diverse datasets reflecting natural emotional expression.
- Real-Time Adjustments: Feedback loops with user confirmation improve accuracy over time.
- Therapist Role: Collaboration with the therapist (e.g., validating AI’s interpretations) can enhance practical outcomes.
In short, AI is helpful but not foolproof in detecting nuanced emotions like those in your example. It currently works best as a supportive tool rather than a standalone detector in high-stakes, nuanced conversations like therapy.
Important Points for Detecting Voice-Based Emotions and Feelings in a Talking AI Mental Health Therapist App …
Detecting voice-based emotions and feelings in a mental health therapy app requires a combination of technical, psychological, and ethical considerations. Here are the key points:
1. Audio Features Extraction
- Pitch and Tone: Variations in frequency can indicate excitement, anger, sadness, or calmness.
- Volume: Loudness changes might suggest intensity or agitation, while softer speech could indicate sadness or hesitation.
- Speech Rate: Faster speech may suggest anxiety or excitement; slower speech can imply sadness or fatigue.
- Pauses and Hesitations: Frequent pauses can reflect uncertainty, distress, or reflective thought.
- Timbre and Voice Quality: Harsh, breathy, or strained voice qualities may suggest specific emotional states.
2. Linguistic Content Analysis
- Word Choice: Use of negative language (e.g., “hopeless,” “useless”) may point to depressive tendencies.
- Sentence Structure: Disorganized speech can indicate anxiety or stress.
- Repetition and Focus: Repetition of certain phrases may signal fixation, anxiety, or stress triggers.
3. Context Awareness
- Situational Relevance: Align tone and emotion detection with the context of the user’s conversation (e.g., discussing a loss vs. daily stressors).
- User History: Incorporate past interactions to personalize detection and interpretation.
Reimagining the Way We Approach Wellness Coaching
“Ask not what others can do for you–ask what you can do to help others.”
- Bill Gates (Microsoft Founder)
- "We should be very careful about artificial intelligence. "AI will eliminate jobs."
- Mark Cuban
- "AI will take over more jobs than it creates, you better be worried."
- Elon Musk
- "Robots will be able to do everything better than us... I mean all of us."
- Sam Altman (Founder of ChatGPT)
- "A lot of jobs that exist today - AI is going to automate those away."
- Oxford University estimates 47% of jobs in the U.S. are at risk of automation
OUR TEAM
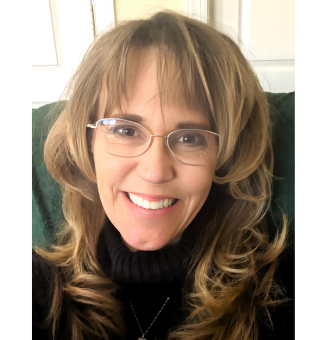
Dr. Denise-Elise Snipes
Director of Mental Health Content
Dr. Dawn-Elise Snipes is a YouTube influencer with over 500,000 followers, a licensed professional counselor, educator, and mental health expert with more than two decades of …
Read more
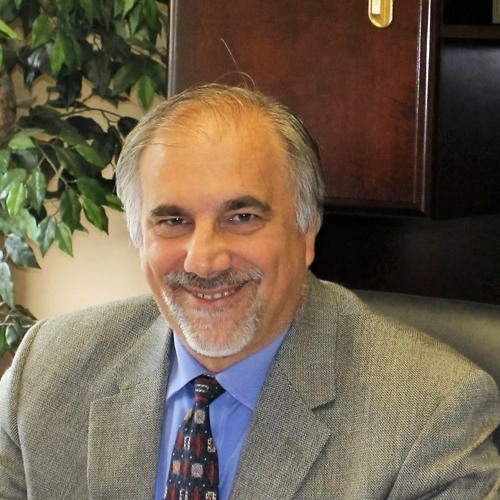
Dr. Joseph Volpicelli
Director of Substance Abuse Content
Dr. Joseph R. Volpicelli is a renowned American psychiatrist and researcher who played a key role in the FDA’s approval of Naltrexone in 1994 for alcohol use disorder (AUD)…
Read more
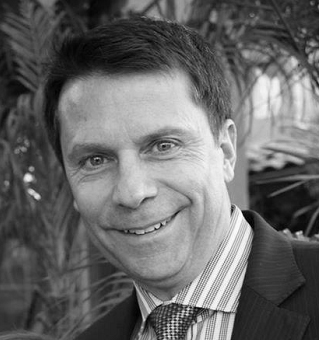
Jay Lacny
Co-Founder & Co-CTO
Jay serves as Co-CTO alongside Professors Milan and Goran. Together, they have successfully developed and deployed similar technologies in various industries.
Read more
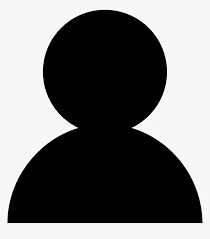
Your Name Goes Here
Director of Sales
We are seeking a Director of Sales with 10+ years of experience and established relationships with ACOs, payors, and self-insured employers in the healthcare industry.
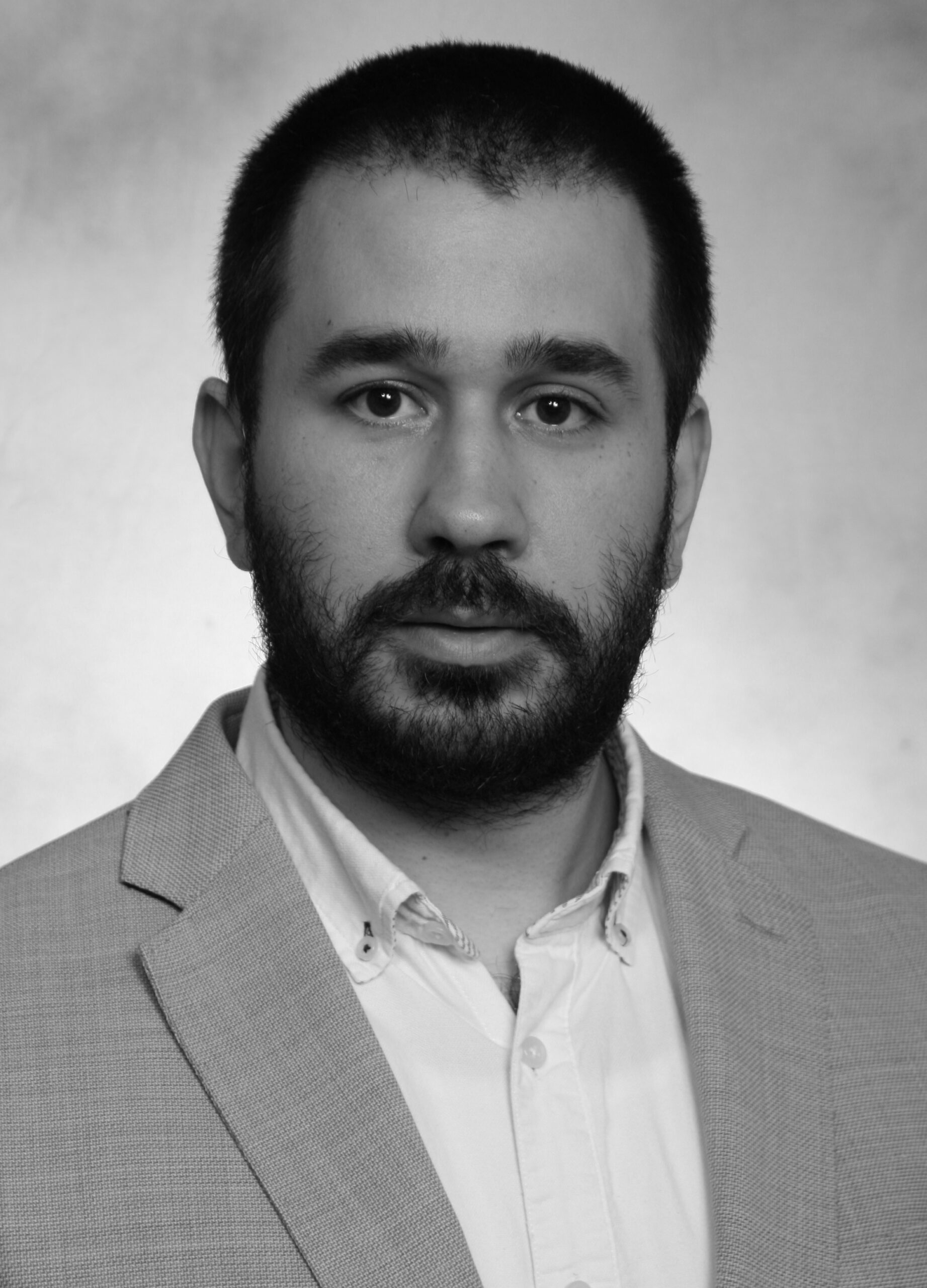
Professor Milan Segedinac, PhD
Chief of Artificial Intelligence
Professor Segedinac is a university professor lecturing & holding practical classes on artificial intelligence, web applications advanced web technologies, knowledge-based systems, and semantic web.
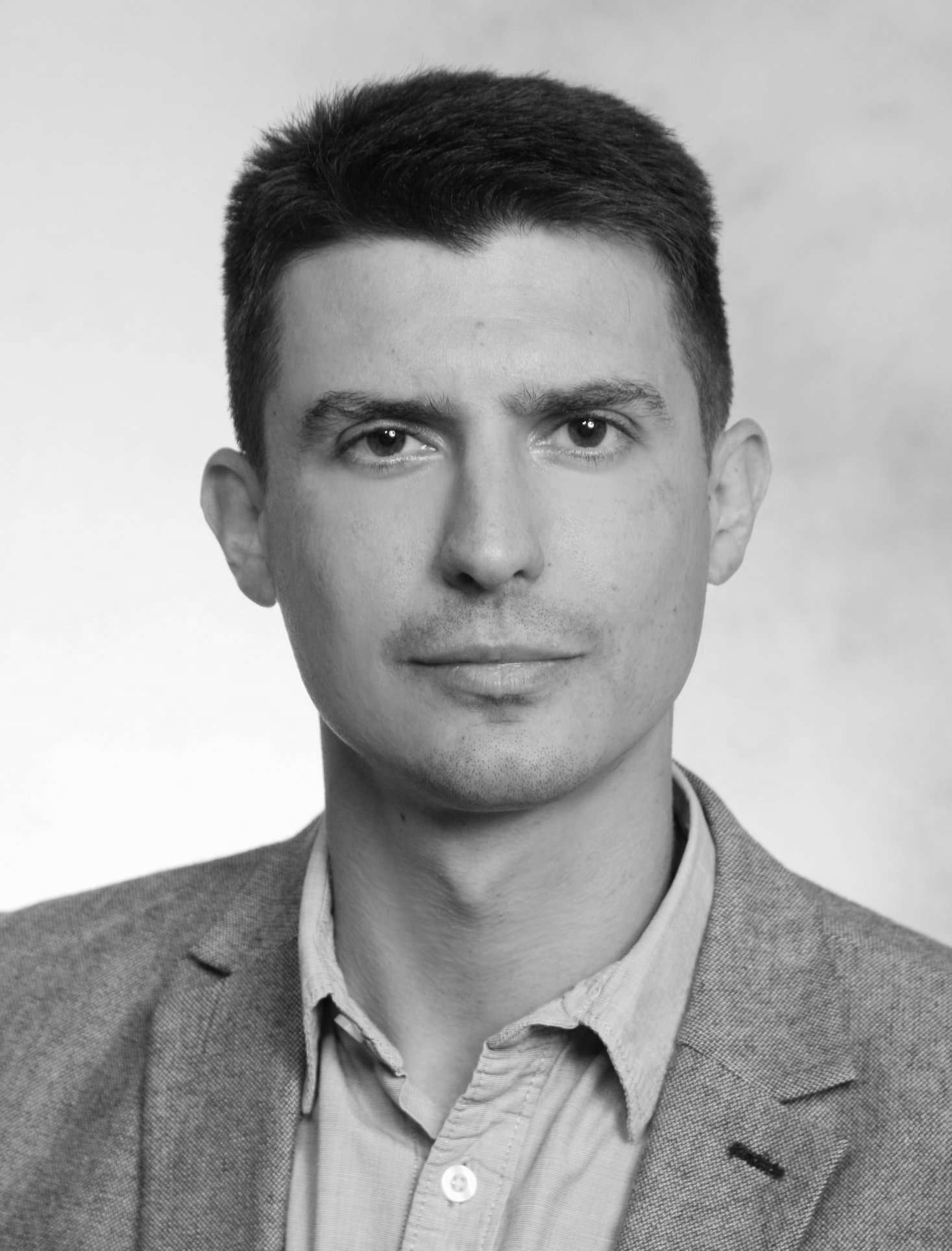
Professor Goran Savić, PhD
Director of Machine Learning
Professor Savić is a university professor, published author, and senior software engineer. He has 10+ years of experience in building machine learning & enterprise information systems.
Read more